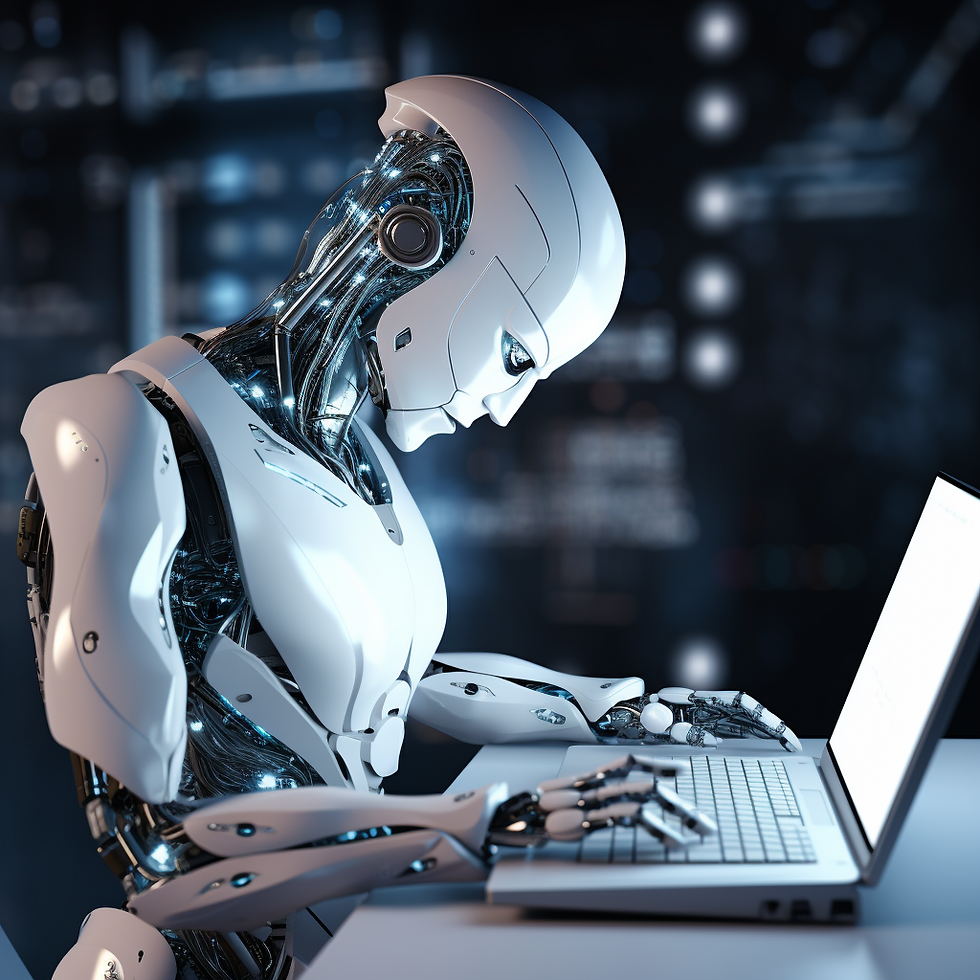
The advent of Vision-Language Models (VLMs) marks a revolutionary stride in artificial intelligence. Merging visual understanding and linguistic processing domains, VLMs represent the cutting edge in enabling machines to interpret and interact with the world in ways akin to human cognition. This guide delves into VLMs' essence, significance, and potential for transforming our interaction with technology.
Understanding Vision-Language Models:
VLMs are sophisticated AI frameworks designed to comprehend and generate content that combines visual elements with natural language. These models are trained on vast datasets of images and text to learn correlations between visual cues and linguistic expressions. By doing so, VLMs can perform various tasks, from describing the content of images in detail to answering complex questions about visual data.
The Significance of VLMs:
The development of VLMs is significant for several reasons. Firstly, they bridge the gap between human and machine perception, offering a more intuitive way for machines to understand and interpret the visual world. Secondly, VLMs have the potential to revolutionize industries by enhancing accessibility, automating content creation, and improving decision-making processes through advanced visual insights.
Key Applications of VLMs:
Accessibility: VLMs can make the Internet more accessible for visually impaired individuals by accurately describing visual content.
Content Creation: In digital marketing and social media, VLMs can automatically generate engaging visual content descriptions, summaries, and captions.
Education and Training: VLMs can offer personalized visual learning experiences, making education more interactive and engaging.
Autonomous Systems: From self-driving cars to drones, VLMs enhance the perceptual capabilities of autonomous systems, enabling them to navigate and interact more effectively with their environment.
How VLMs Work:
At their core, VLMs integrate two main components: a vision and language subsystem. The vision subsystem processes and understands images using convolutional neural networks (CNNs) or transformers. The language subsystem, often based on transformers like GPT (Generative Pre-trained Transformer), processes and generates natural language. The interaction between these components allows VLMs to understand and describe visual content accurately.
Challenges and Future Directions:
While VLMs hold immense potential, they also face challenges, including data bias, ethical considerations, and the need for computational efficiency. Future advancements are expected to address these challenges, making VLMs more adaptable, moral, and accessible for broader applications.
Conclusion:
Vision-language models are poised to redefine our interaction with technology, offering new ways to engage with digital content, automate tasks, and enhance decision-making. As VLMs evolve, they promise to unlock unprecedented possibilities across various sectors, making the world more connected, accessible, and intelligible.
Commentaires