Empowering Customer Engagement: The Role of Machine Learning in Customer Segmentation and Communication Scheduling
- AV Design Studio
- Mar 6, 2024
- 3 min read
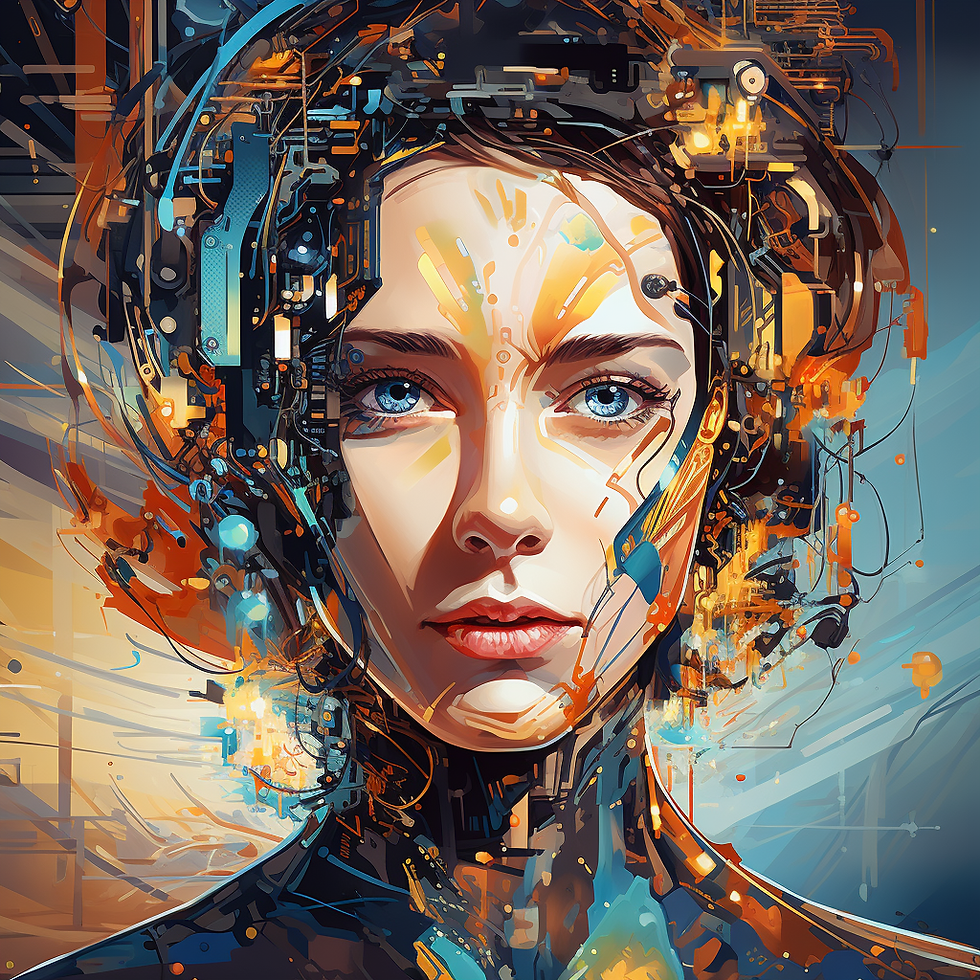
In the age of digital transformation, personalization and precision in customer engagement strategies have transitioned from being a luxury to a necessity. Traditional segmentation and communication planning methods fall short as businesses strive to understand and cater to their customers' increasingly diverse and dynamic needs. Enter Machine Learning (ML), a revolutionary technology that's changing the game by enabling businesses to segment customers more effectively and plan personalized communication schedules at an unprecedented scale. This blog post delves into how ML can transform customer engagement strategies, from segmentation to scheduling communications like emails and SMS, ensuring each interaction is timely, relevant, and, most importantly, welcomed.
The Power of Machine Learning in Customer Segmentation
Customer segmentation divides a customer base into groups of individuals similar in specific marketing-related ways, such as age, gender, interests, and spending habits. While traditional segmentation methods rely on broad categories and assumptions, ML introduces granularity and accuracy that was previously unattainable.
1. Advanced Data Analysis: ML algorithms can process vast amounts of data, identifying patterns and correlations that humans might miss. This ability allows for creating particular customer segments based on various attributes, including behavioral data, purchasing history, interaction preferences, and sentiment analysis derived from social media and customer feedback.
2. Dynamic Segmentation: Unlike static segmentation models, ML enables dynamic segmentation, where customer groups are continuously updated as new data comes in. This ensures marketing strategies remain relevant and aligned with changing customer behaviors and preferences.
3. Predictive Insights: ML segments customers based on past and present behaviors and predicts future actions. This predictive capability allows businesses to anticipate needs, preferences, and potential churn, enabling proactive engagement and personalized offerings.
Scheduling Communications with Precision
Once customers are segmented effectively, the next challenge is determining what to communicate, how, and when. ML transforms this aspect of customer engagement by analyzing customer data to inform the content of communications and the timing and channel preferences for each segment.
1. Personalized Content Creation: By understanding each segment's unique characteristics and preferences, ML can help tailor the content of emails and SMS messages to match. Whether highlighting products of interest, addressing specific concerns, or offering relevant rewards, ML-driven content personalization ensures higher engagement rates.
2. Optimal Timing and Frequency: One of the most significant advantages of using ML is determining the best time to send communications to each customer segment. By analyzing engagement patterns, ML algorithms can predict when customers are most likely to open, read, and act on emails or SMS messages. This optimization extends to determining the ideal frequency of communication, balancing engagement with the risk of customer fatigue.
3. Channel Preference: Not all customers engage with communications through the same channels. ML helps identify whether email, SMS, social media, or another channel is the most effective way to reach each segment, enhancing the likelihood of successful engagement.
4. Automated Scheduling: ML algorithms can automate communications scheduling, ensuring that messages are sent at the optimal time and through the preferred channel for each customer. This automation increases efficiency and allows real-time adjustments based on ongoing data analysis.
Implementing ML in Customer Engagement Strategies
While the potential of ML in customer segmentation and communication scheduling is vast, effective implementation requires a strategic approach:
1. Data Quality and Integration: The accuracy and effectiveness of ML algorithms depend on the quality and comprehensiveness of the data they process. Businesses must ensure that data from various sources is clean, well-organized, and integrated to provide a holistic view of the customer.
2. Choosing the Right Algorithms: There is no one-size-fits-all ML algorithm for customer segmentation and communication planning. Businesses must experiment with different algorithms, customize them according to their specific needs, and continuously refine them based on performance.
3. Privacy and Compliance: As businesses leverage personal customer data to fuel their ML models, they must diligently navigate privacy concerns and regulatory compliance issues. Transparent communication about data use, securing customer consent, and implementing robust data protection measures are critical.
4. Continuous Monitoring and Optimization: ML-driven customer engagement strategies should not be set and forgotten. Continuous monitoring of performance metrics and regular optimization of algorithms and techniques are essential to staying ahead in a rapidly changing market.
Conclusion
Machine Learning is redefining the landscape of customer engagement, offering businesses unprecedented capabilities to segment their customers and schedule personalized communications efficiently and precisely. By leveraging the insights and automation capabilities of ML, companies can ensure that every interaction with their customers is timely, relevant, and tailored to individual preferences, thereby enhancing engagement, loyalty, and, ultimately, business success. As we look to the future, integrating ML into customer engagement strategies will no longer be an option but necessary for businesses aiming to thrive in the competitive digital marketplace.
Comments